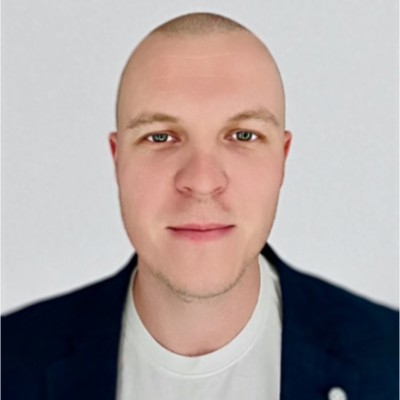
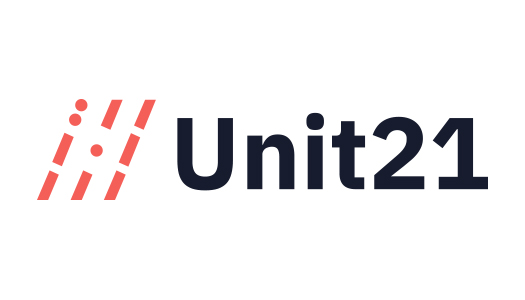
Remember the movie "Catch Me If You Can," where Tom Hanks' character meticulously combines clues to track down the elusive Frank Abagnale Jr? Tom Hanks' character was manually piecing together the best data points like address and telephone to track down his fraudster.
Fraud today is still often a game of cat and mouse, and top fraud fighters find that some of the data points that were most effective then, like address, phone number, etc., have high match rates to fraudsters today. A recent study revealed that by harnessing precise data points like phone numbers and addresses, true positive rates in fraud detection can soar to over 90%. Let's look at some of the top data points, their true positive rates, and how graph analysis (graph-based rules) combined with impactful data points can help you move to fraud prevention.
Harnessing Data for Advanced Fraud Prevention
This is our fifth installment in Fraud Files, our blog series that shares unique insights based on data from over 4.05B events on the Unit21 platform. In our previous posts, we explored several trends and insights, including:
- Part 1: The rate of true positives for fraudulent events for accounts in their first 90 days
- Part 2: The differences for banks and credit unions on fraud activity detected in their first 14 days
- Part 3: Why keeping an eye on account dormancy is as essential as monitoring account action
- Part 4: A look into recent fraud trends by top rules created
The Dramatic Rise of Rule Creation in 2023-Q2
Our data shows a similar trend–phone number and physical address matches stand out at the forefront, with true positive rates of 95.21% and 85.02%, respectively. These figures are more than just statistics; they represent the frontline defense in identifying and halting fraudulent activities. The efficacy of these matches cannot be overstated, as they are highly reliable indicators for identifying legitimate instances of fraudulent activity when they are accurately collected and verified.
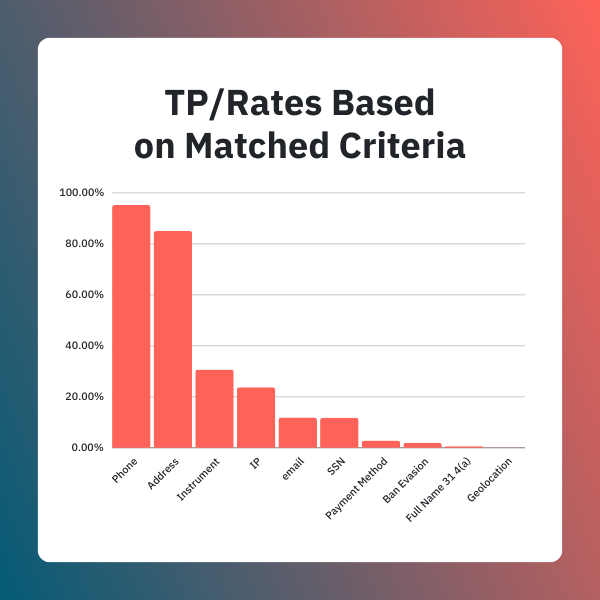
However, the match criteria landscape is far from uniform. A deeper dive into the chart reveals significant variability in performance across different data points. Unlike the high success rates of phone and address matches, others lag.
Full names and socials have low match rates. Common reasons can include the great availability of compromised and synthetic identities – which allow the bad actors to operate several entities on one financial platform, commonality and variability of names, and data quality issues. Relying on match rates around these standalone points can be tricky. Digital match criteria such as IP and email have mid-range true positive or fraud match rates and present significant challenges in fraud detection, as IP and email addresses can easily be altered or masked, obscuring the fraudster's actual digital footprint. Furthermore, these digital identifiers often need more contextual information to differentiate between legitimate and fraudulent activity accurately, compounding the difficulty of pinpointing suspicious transactions.
Obviously, relying on a single data point is never an effective strategy, but piecing all the data together is a complex puzzle that requires both breadth and depth in data analysis. For example, we had a client combine last names and dates of birth to generate an impressive 92% true positive rate for fraud detection. This example illustrates how pairing seemingly weak individual signals can create a powerful signal together.
The Power of Graph-Based Analysis in Fraud Prevention
So, how do you piece it all together? Graph-based analysis, or as we call it at Unit21, Graph-Based Rules, is a sophisticated approach that transcends traditional analysis methods by mapping out the intricate web of relationships and connections that exist between transactions, accounts, and users.
Graph-Based Rules stand out for their ability to visually connect the dots across what might appear to be unrelated information. For example, a bank can flag multiple accounts linked to a single social security number, to uncover a sophisticated scheme where fraudsters create duplicate accounts to exploit sign-up bonuses. Graph-Based Rules unveil the complex networks underpinning sophisticated fraud schemes such as money laundering, synthetic identity fraud, fraud rings, and network-based fraud. Using Graph-Based Rules, fraud detection teams can navigate the convoluted paths that fraudsters tread.
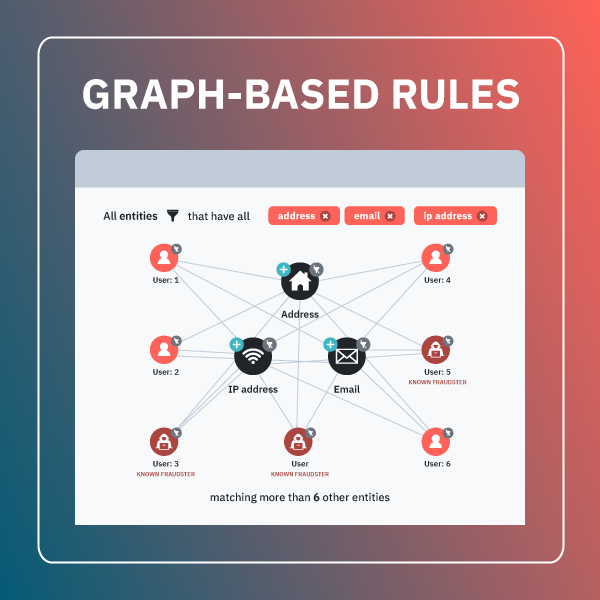
The Takeaway
Deploying a blend of high-accuracy match criteria like phone numbers and addresses with the expansive network insights offered by graph-based rules gives the agent a visual advantage in studying the legitimacy of connections between users. In the spirit of "Catch Me If You Can," it's not just about chasing shadows; it's about illuminating the entire network, ensuring no stone is left unturned in the quest to protect your institution. This holistic approach marks a pivotal shift in fraud prevention, heralding a future where security and precision walk hand in hand. Want to learn more about leveraging data points with high true positives and Graph-Based Rules? Join our upcoming Graph-Based Rules workshop or book a demo.
Subscribe to our Blog!
Please fill out the form below: